Improving biodiversity protection through artificial intelligence
Silvestro, D., Goria, S., Sterner, T., and Antonelli, A. (2022) Nature Sustainability, DOI:10.1038/s41893-022-00851-6
Download open access article: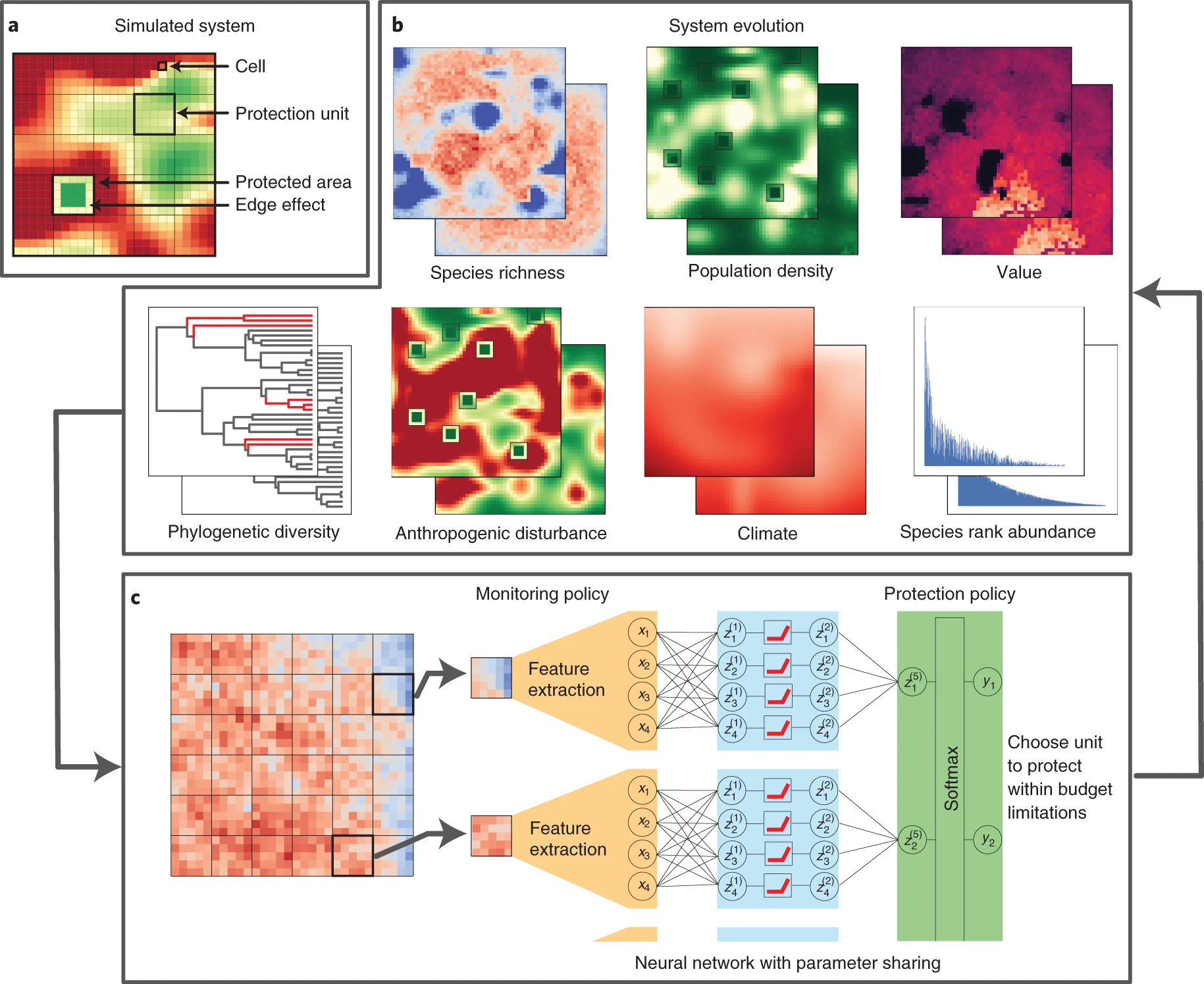
Using artificial intelligence to optimize ecological restoration for climate and biodiversity
Silvestro, D., Goria, S., Groom, B., Sterner, T., and Antonelli, A. (2025) bioRxiv, DOI:10.1101/2025.01.31.635975
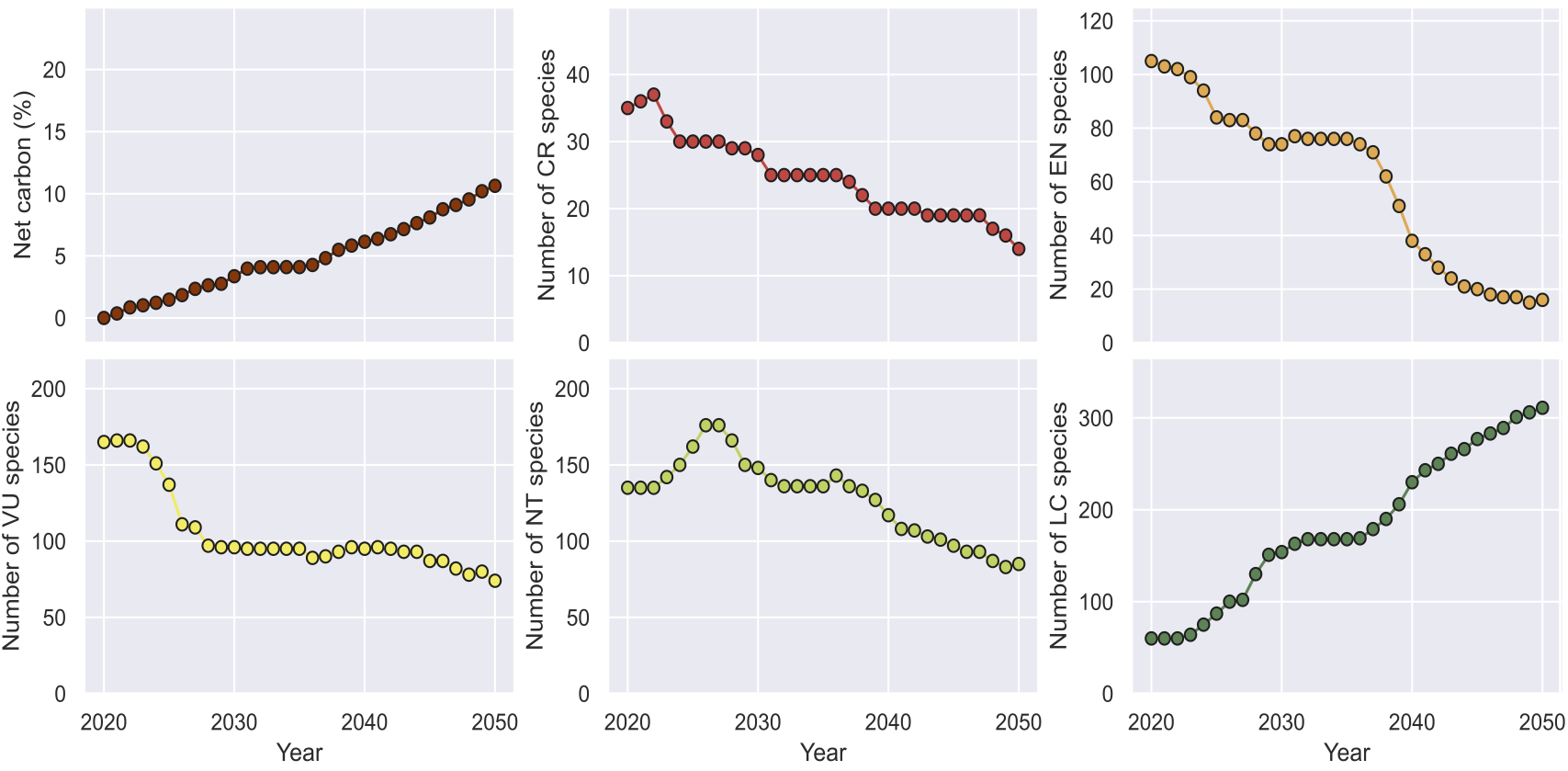